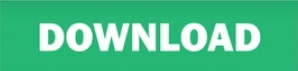
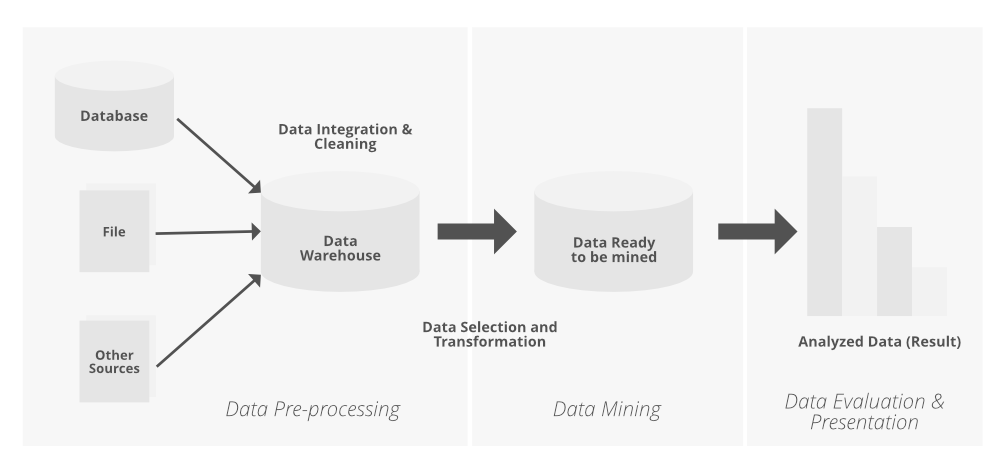

The severe testing account leads to a reformulation of statistical significance tests: Moving away from a binary interpretation, we test several discrepancies from any reference hypothesis and report those well or poorly warranted. A claim can be probable or even known to be true, yet poorly probed by the data and model at hand. When a test’s error probabilities quantify the capacity of tests to probe errors in C, I argue, they can be used to assess what has been learned from the data about C. This probability is the severity with which C has passed the test. I put forward a general principle for evidence: an error-prone claim C is warranted to the extent it has been subjected to, and passes, an analysis that very probably would have found evidence of flaws in C just if they are present. Can a method's error probabilities both control a method's performance as well as give a relevant epistemological assessment of what can be learned from data? As reforms to methodology are being debated, constructed or (in some cases) abandoned, the time is ripe to bring the perspectives of philosophers of science (Glymour, Mayo, Mayo-Wilson) and statisticians (Berger, Thornton) to reflect on these questions.Can we use the same data to search non-experimental data for causal relationships and also to reliably test them?.How should we cope with the fact that data-driven processes, multiplicity and selection effects can invalidate a method's control of error probabilities?.
#Data dredging how to
Experts do not agree on how to improve reliability, and these disagreements reflect philosophical battles–old and new– about the nature of inductive-statistical evidence and the roles of probability in statistical inference. Benedum PSA 2022 powered methods, the big data revolution, and the crisis of replication in medicine and social sciences have prompted new reflections and debates in both statistics and philosophy about the role of traditional statistical methodology in current science. High powered methods, the big data revolution, and the crisis of replication in medicine and social sciences have prompted new reflections and debates in both statistics and philosophy about the role of traditional statistical methodology in current science.
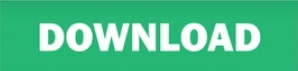